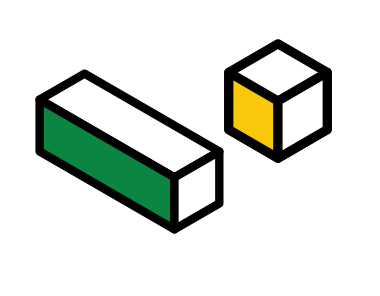
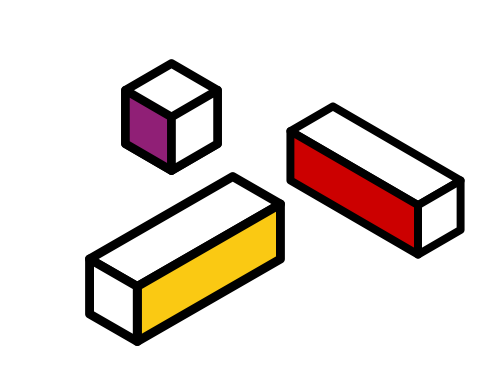
B2C eCommerce: Know Your Customers, Including Those You'll Never Meet
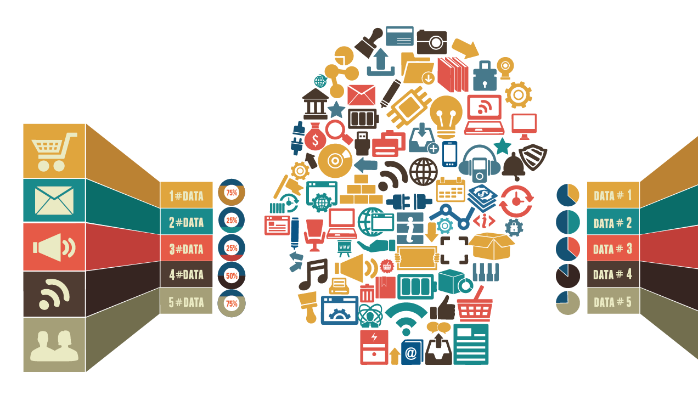
In the eCommerce space, businesses rarely meet their customers. However, they often have the most data about their customers, such as transactions, website movement, shopping cart abandonment, and more.
Just what can eCommerce sites do with this treasure trove of customer information?
Use it to maximise profits, of course!
Let's talk about top global eCommerce marketplaces, like Ebay. They use customer behavior patterns to increase the likelihood of making a sale. Chinese giant, Alibaba, uses customer data collected on its platform to develop a Credit Scoring Service and gauge users' creditworthiness when offering them financial services.
In this blog post, we share the benefits of Knowing Your Customer in the B2C eCommerce space. [ed note: This is based on our Know Your Customers Framework written up in an earlier post.]
The experiment: eCommerce customer segments & other insights
In the E-Commerce environment, customers are typically required to sign into an account, find the item they would like to buy, and then execute the purchase(s). These activities provide 3 main types of data:
- Account Login (Demographic)
- Find item on the site (Behavioral)
- Execute the purchase (Transactional)
I used an E-Commerce transactional dataset of a gift shop in UK (Ecommerce dataset from Kaggle), and a data visualiser (Tableau) to demonstrate how eCommerce websites use data analytics to extract customer insights.
The actual data analytics procedure is quite lengthy. So we've turned it into a nifty handbook titled: "How We Do It: Data Mining Transactions to Know Our Customers" for you to download. It contains how we did our data mining and what we found. It's a useful primer for eCommerce owners who are new to data analytics.
We'll explain our findings in the rest of this post.
Customer segments: RFM & insights
Using a simple RFM ("Recency, Frequency, Monetary") Framework, we developed the following customer segments.
Based on the RFM framework, we could determine how much and how recently and frequently customers have been spending, to group them accordingly.
Below, we evaluate the proportion of our 7 identified customer segments.
It seems like there are 4 big groups and 3 smaller groups.
Insight 1: Targeting the bigger groups would increase our spending as their numbers are bigger. The opposite can be said for the smaller groups.
Perhaps, we should observe the customer segment that generates the most revenue first.
The graph clearly shows that “Good Customers” (Buy frequently, generate a good amount) created the most revenue for the organisation. This is in line with Pareto's principle: “For many events, roughly 80% of the effects come from 20% of the causes.”
Insight 2: "Good Customers" are this organisation's primary revenue generators.
Take action based on these 2 insights
With this information, we know that we can generate the most returns by targetting "Good Customers." However, we shouldn't neglect the other customers. Let's diversify some marketing efforts on other customer segments, whilst focusing on "Good Customers."
- Champion Customers: Get them returning and remembering us. Provide gift cards as tokens of appreciation.
- Frequent Customers: They transact often but do not spend a lot. Let's find out why by conducting a survey.
- Good Customers: Find other ways to shower attention and love for our key customer segment [Wen Wei: we love these guys—they generate the most revenue!]. Let's look at their web behaviours to understand their purchase flow to provide rewards and ease of use.
- Infrequent Customers (Low Value): These guys neither transact often nor spend much. Perhaps they are displeased with our products. We could examine their product purchases to find their least favoured product combinations, and modify from there.
- Infrequent Customers (High Value): These guys don't transact often but when they do, they are willing to spend. Might it be time- or topic-related? We could conduct a time-related mini experiment (such as our Dynamic Time Warping Experiment).
- Lost Customers (Low Value): They have not transacted for a very long time and even when they did, they did not spend much. It's probably safe to ignore them as their digital marketing ROI may not even break even.
- Lost Customers (High Value): They last transacted quite some time ago, but they were willing to spend. It's rather murky why they're doing this: are they now buying from our competitors? Or have they simply moved away? Or maybe they've decided on another type of product? We could run surveys and sentiment analyse their social media feeds to find out what happened.
Additional insights: Best customer, top-selling items, and best timings
It is quite natural to unearth other insights while enroute to customer segment discovery. Here are some insights I discovered for this dataset:
Best customer is 17450
We should strengthen the relationship with Customer ID 17450. We should find out our business relationships and more information to unearth opportunities with this customer.
Most bought items by customers in a time period
Customer ID 14096 and Customer ID 17841 are buying quite a number of items at specific time periods. Why? What's causing it? If we can unearth the reasons for these patterns, we could identify a niche group of customer segments that weren't immediately apparent.
Customers who stopped buying from us
We need to look at the list of customers on the right and identify possible reasons for them not purchasing from us anymore.
Best product & customer relationship
Item 23843 is our bestseller and only Customer ID 16446 is buying them. We definitely have to treat them right. We should investigate this business relationship and identify other areas where we could assist them. The same can be said of Item 23166 and Customer ID 12346.
Items producing the most revenue
The smaller items are generating the most revenue. Perhaps we should R&D more of these items and produce more of them.
People are buying in the hours of...
We could place our digital marketing focus during office hours and possibly on weekdays to gain the most exposure.
Data can help you know your customer
Hopefully, we’ve shown how Knowing your Customers can generate insights that could be valuable in your business. It's just a matter of getting the right dataset and enough data, and knowing what to do—which we've hopefully shown in this blog post!
Have you tried analysing your eCommerce or transaction dataset? What nuggets of interesting insights did you unearth? We'd love to hear from you!
Read: Every eCommerce Resource You’ll Ever Need to Set Up Your Online Store in Singapore
Data@Construct: Forge Better Decisions
We run weekly Data Science Experiments to help marketers use data to increase their Digital Marketing ROI and Effectiveness. Sometimes we’ll do wacky stuff, sometimes we’ll focus on common business and marketing problems, but always, we’ll share our learnings here on our blog.
Please subscribe to our Email Updates for weekly updates and learnings (or just head over to our Big List of Data Experiments). If you’ve got a marketing data problem that needs cracking, we’d love to hear from you.
More insights