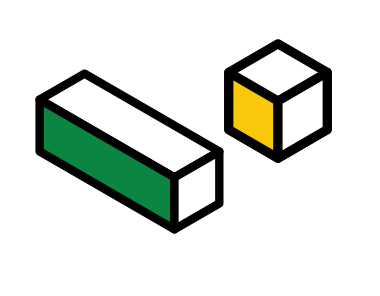
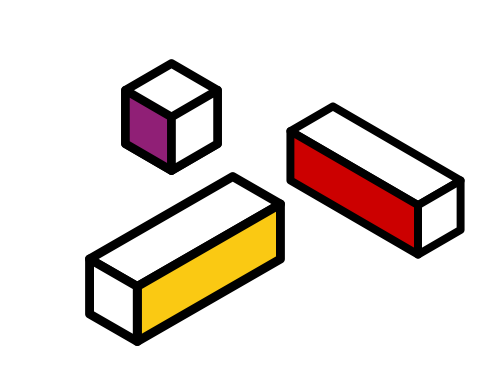
An Executive POV to Understanding Data Analytics: How Descriptive vs Predictive vs Prescriptive Analytics can drive your Business Transformation
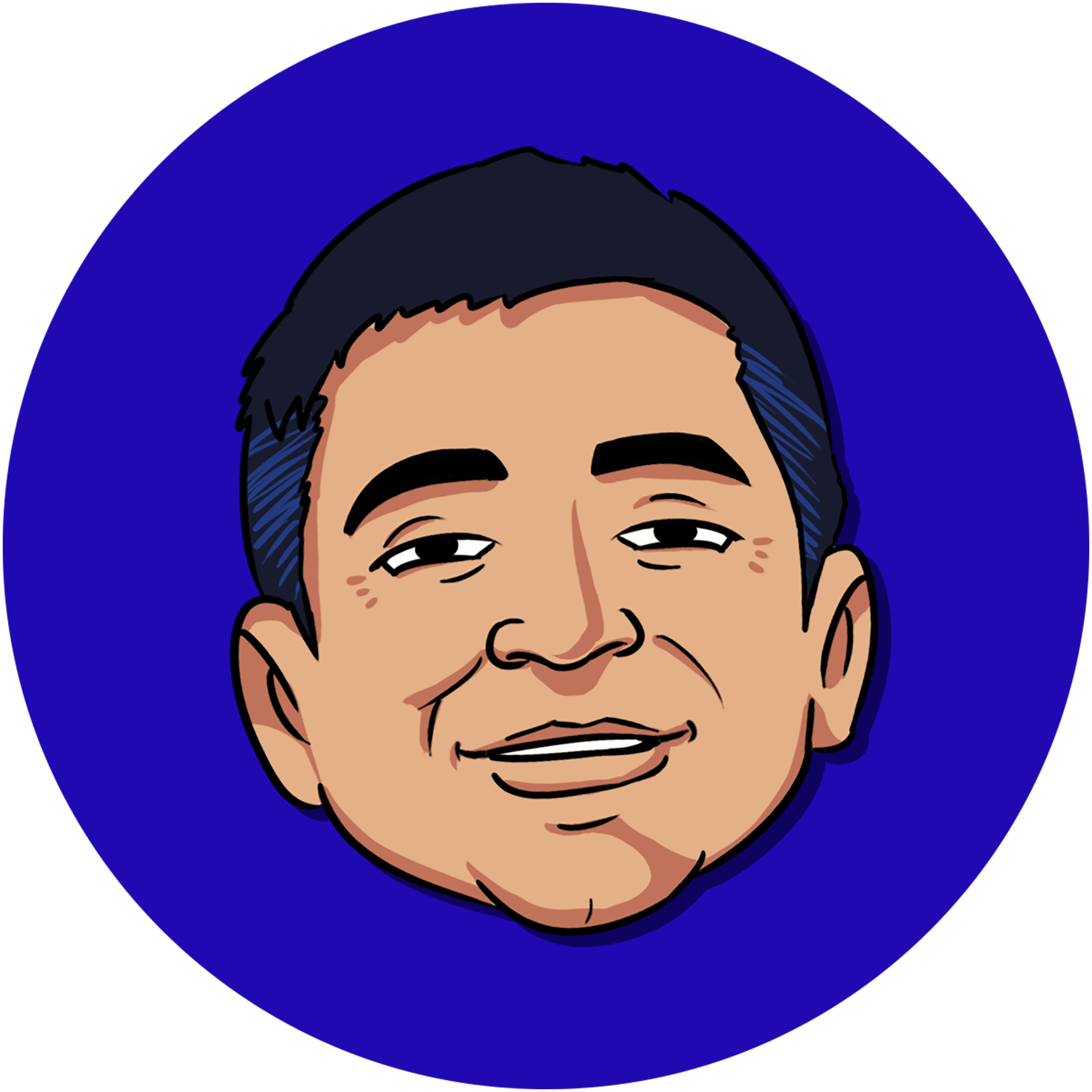
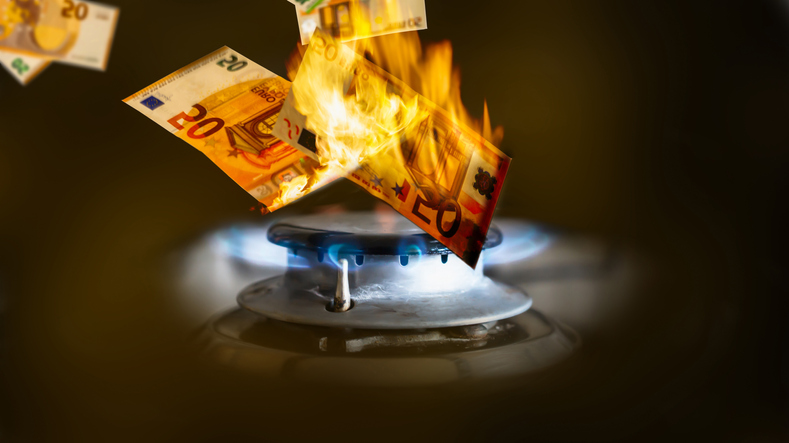
How much data does your company generate? What kind of analytics should you perform? Should you even bother analysing data at all?
Data analytics has become a critical part of modern business operations with companies generating massive amounts of data every day. This data is being collected from various sources such as customer interactions, social media posts, web logs, etc. The challenge lies in understanding this data and extracting actionable insights.
Analytics is the science of deriving knowledge from data. There are three types of analytics: descriptive, predictive, and prescriptive. Each type of analysis provides a unique perspective into the data and helps companies derive meaningful insights that can drive Business Transformation.
How is Analytics Transforming Businesses Today?
Technology serves as an enabler for real-time business transformation – analytics technology makes enterprises more agile in the matter of maintaining pace with ever-changing customer needs and business trends.
The big data innovators today use analytical tools for high-level forecasting as well as real-time visualisation. Businesses that embrace analytics technology transform their business models, and encounter new opportunities for customers, products, revenue streams, and services. From sourcing materials and forecasting demand to the accounting and human resource activities, every aspect of the business can be with analytics.
Why Data Analytics is Key to Digital Transformation
From our experience working with Mid-sized companies in Asia, information has not yet become an established part of most companies' business models. It remains at least somewhat new. Therefore, it continues to be a valuable source of differentiation among leading organisations.
Data and Analytics must become Strategic Priorities.
Data and analytics are crucial for digital transformation initiatives. Today, less than half (45%) of documented corporate strategy mentions data and analytics as fundamental elements for driving business performance.
With the ability to develop significant competitive advantage, data and analytics must become a strategic priority for your digital transformation roadmap.
Why is Data Analytics so Hard? Challenges faced in adopting a Data And Analytics Strategy
Data has the potential to transform business in profound ways, but Data and Analytics are not easy. Data is messy, Data is diverse, Data is unstructured, Data comes with its own problems.
Data infrastructure issues can cause multiple problems for businesses. Data sources may be inconsistent across internal data silos or external systems due to lack of integration between systems. We look at some of the main issues faced by Businesses when trying to adopt a data-led strategy.
Data Silos
Gathering high quality data can be problematic as department heads have hoarded data for decades in hard to access excel spreadsheets and the IT applications which have often been developed to meet specific departmental needs don't communicate well with one another. Moreover, bridging data silos is difficult as such initiatives tend to rely on the IT department, which often has other more pressing priorities. Also, doing the analysis takes time and it's quite complicated.
Data Maturity
IDC has defined five stages to identify the maturity of an organization with regard to its big data undertakings as well as the steps it can take in order to mature further.
Ad Hoc: Data initiatives are driven as and when a business requirement arises. There is an absence of a specific analytics deployment model, individual roles are not properly defined, and there is a lack of a measured approach to resource allocation.
Opportunistic: There is a clearer understanding of the business goal. However, there still exist inefficiencies in project management and resource allocation.
Repeatable: Data Analytics strategies are defined for various business undertakings. Also, there is sufficient budget allocation and stakeholder buy-in.
Managed: Analytics deployment models and data strategies are more aligned with corporate objectives. Best practices are determined, and substantial ROI is consistently achieved through the methods in use. Data-driven organisations turn data into a strategic asset, and analytics becomes an essential component of its operational DNA.
Optimised: The company has established a solid data and analytics infrastructure. There is a highly effective center of excellence in charge of these projects. To achieve significant business value and impact, the organization uses disruptive deployment methods and tools. The objective now is to foster innovation and continuous corporate change in order to gain unrivalled business value and impact.
The IDC survey included 1,810 organisations. Only 59 of the respondents were classified as "advanced" in terms of big data maturity, according to IDC. In terms of the maturity framework described above, a significant proportion of the innovators are in the managed stage, while others are getting closer to crossing the repeatable stage and a few are still in the Ad Hoc Stage.
Talent Deficit
There is a lack of Talent currently within organisation who can wrestle with vast amounts of data to make sense of it. Data Scientists are in short supply, Data Analysts are needed to interpret the data, Data Visualisation experts are required to communicate insights. Without a clear strategy, it will be difficult for executive to justify the large investments needed to develop their organisations's Data Maturity.
Tools Deficit
A rich mix of Data Management, Data Warehousing and Analytics tools are available in the market that can yield insights when used effectively and where appropriate. However, there is a dearth of tools to manage Data Discovery, Data Analytics and Data Visualisation, which are critical to get the right insights at the right time. Again these require significant investments, so Executives will need to build a clear Roadmap and business case to justify such investments.
Lack of executive Sponsorship
Adopting a transformative data strategy is hard because of the lack of understanding of what Data Analytics is and how it can be applied to drive value in a business.
Most executives acknowledge the importance of data in digital transformation, but when it comes to their own decision making, they are more likely to make intuition and gut feel driven decisions. After all, it's their many years of experience that has landed them in their position of authority isn't it? Also, The amount of patience needed to overcome the challenges of data transparency and the patience needed in waiting for the time it takes to carry out analytics are not commonly observed traits of typical executive behaviour. While there is no one universal recipe, paying attention to organisational alignment, cross functional business processes, and executive education is likely to improve the odds of success.
Types of Analytics Businesses can Leverage
In order to get the most of analytics for Data Transformation, its important for Senior Executives to understand the types of Analytics available today.
Descriptive Analytics:
This type of analytics helps businesses understand trends and patterns in data. It also provides insights into customer preferences and behaviours.
Most statistical analyses involve counting things, aggregating them, filtering out some of them, applying simple mathematics to them, and then presenting results. There are an infinite number of ways to look at any given statistic. Descriptive Statistics are helpful for showing things like total stock in inventories, average dollar amounts spent by each customer, and yearly changes in sales. Examples of descriptive analysis include reports that provide historical insight regarding the company’s finances, operations, sales, etc.
Three basic cornerstones of predictive analytics are:
- 1) Predictive modelling
- 2) Decision Analysis and Optimization
- 3) Transaction Profiling
When you need to understand at a summary level what’s happening within your organization, use descriptive analytics. And when you want to explain specific details about your business, use predictive analytics instead.
Predictive Analytics:
This type helps predict future events and outcomes based on past performance. For example, if you know that customers tend to purchase products from your store during the last week of the month, then you could send an email reminder to those customers about upcoming sales. This type of Analytics answer: “What could happen?”
A commonly used example of predictive analysis is using it to generate a credit score. Financial institutions use these scores to determine whether their customers are likely to pay back loans they've taken out. Businesses use predictive analytics for various purposes including understanding how sales might close during the year, predicting which products people may buy together, or forecasting inventory needs based upon a multitude of factors.
Prescriptive Analytics:
This type aims to help companies make better decisions by providing recommendations for actions or changes. These types of analytics can include machine learning algorithms. Optimization and simulation algorithms to advise on possible outcomes and answer: “What should we do?”
Prescriptive analytics are relatively complex to administer, and most companies are not yet using them in their daily course of business. When implemented correctly, they can have a large impact on how businesses make decisions, and on the company’s bottom line. Larger companies are successfully using prescriptive analytics to optimise production, scheduling and inventory in the supply chain to make sure they are delivering the right products at the right time and optimising the customer experience.
For instance, an example would be electricity and gas. Prices for natural gas vary greatly from day to day due to fluctuations in supply, demand, economics, geopolitics, and weather conditions. Energy traders, pipeline operators, and energy utilities have an interest in better forecasting natural gas prices because these entities want to be able to hedge their risks by locking in favourable pricing arrangements when they need them most. Prescriptive analytics can help companies forecast future demand for products and services. It can also be used to determine which price point would yield the best profit margin.
In Conclusion
Data and Analytics are strategic assets that help companies transform. The power lies in the ability of the Leadership teams to identify the use cases and types of Analytics that can be most instrumental in business transformation.Information is the strategic asset that will help give your business a competitive advantage. We hope we have given you, the mid-sized business Executive, some food for thought as you drive your companies business transformation by leveraging your business Data.
More insights